These days, artificial intelligence (AI) is more than just a buzzword. It’s a critical tool for enhancing efficiency, driving innovation, and gaining a competitive edge. However, one factor remains essential for the success of your AI initiatives: data quality. Without accurate, reliable, and well-structured data, even the most advanced AI models will fail to deliver meaningful results.
At the heart of every AI initiative lies data. Whether you're automating business processes, enhancing decision-making, or creating predictive models, the success of these projects depends on the foundation of high-quality data. In this article, we will explore why data quality is crucial to AI success and how organizations can ensure their AI initiatives are set up for success.
Data Quality Drives the Performance of Your AI Initiatives
The performance of AI models directly correlates with the quality of the data used for training. Poor-quality data can introduce errors, biases, and inconsistencies, undermining the AI system's integrity. On the other hand, high-quality data enables the AI model to learn accurately and make reliable predictions. By focusing on data quality, organizations can improve the accuracy and reliability of their AI-driven processes.
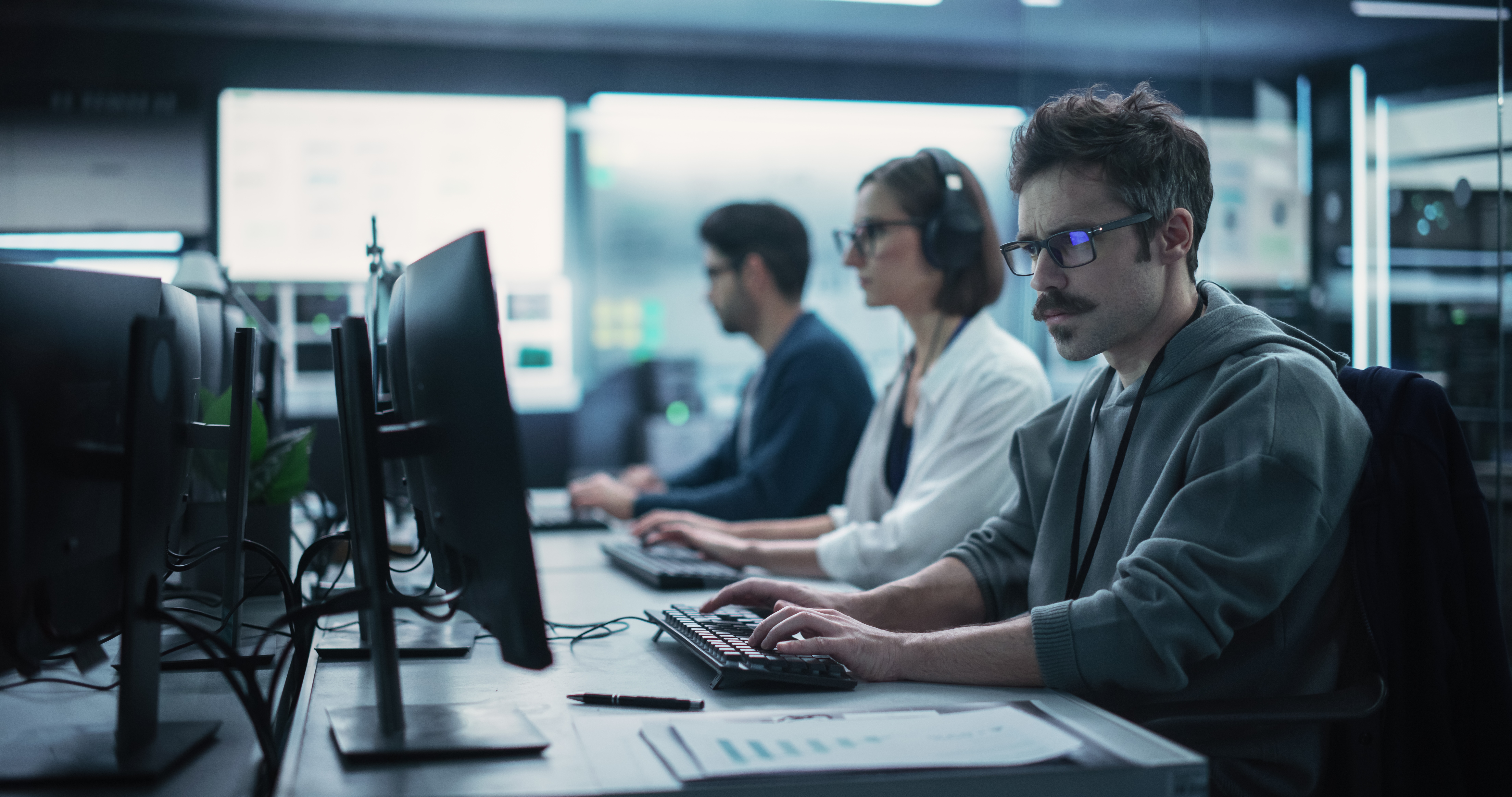
AI models are only as good as the data fed into them. If the data is obsolete or incomplete, the AI system may make flawed decisions that harm business operations. Quality data, however, ensures that the algorithms powering AI systems are robust, efficient, and capable of learning in real time. This, in turn, leads to more insightful outputs and business-relevant outcomes.
For AI initiatives to succeed, data must undergo thorough cleaning, normalization, and validation. Data must also be free from errors, inconsistencies, and duplicates to ensure AI models perform optimally. Therefore, investing in data quality management processes is crucial for getting the most out of AI technologies.
Data Quality Ensures Trust and Compliance
Organizations must adhere to data privacy and compliance standards when implementing AI initiatives. With regulations such as GDPR and CCPA, maintaining the integrity and security of data is not just a best practice—it’s a legal requirement. By ensuring data quality, companies reduce the risk of non-compliance, which can lead to significant penalties and reputational damage.
Moreover, ensuring data quality fosters trust both internally and externally. Employees, stakeholders, and customers need confidence that AI systems use accurate and ethical data to make decisions. Transparency in data processes ensures that AI initiatives are both reliable and trustworthy.
Ensuring data quality is imperative when building compliance into AI initiatives. By following best practices for data governance, organizations can reduce risks and ensure that their AI systems remain compliant with legal and ethical standards.
The Role of Data Governance in the Success of Your AI Initiative
Effective data governance is key to ensuring data quality in AI initiatives. Data governance frameworks establish rules and procedures for data collection, storage, access, and usage. By having a clear governance strategy, organizations can maintain high data quality, consistency, and security, which are necessary for AI models to function at their best.
A comprehensive data governance program ensures that all stakeholders are on the same page regarding how data is handled and used. This is particularly important for AI initiatives that require seamless integration with multiple data sources and platforms. By setting clear data management policies, organizations can avoid issues such as data silos and inconsistencies, which can negatively affect AI performance.
Organizations looking to implement AI initiatives should invest in data governance tools that automate and streamline data management. This makes it easier to maintain data quality, track data usage, and ensure compliance with regulatory standards. Well-executed data governance practices create a stable foundation for AI to thrive.
Data Accessibility and Integration for AI Success
Data integration is a significant challenge in AI initiatives, as organizations often rely on disparate data sources stored in various formats. For AI systems to function effectively, they require data from multiple departments and systems to be accessible, well-structured, and integrated. This means that data must be organized and linked across the organization.
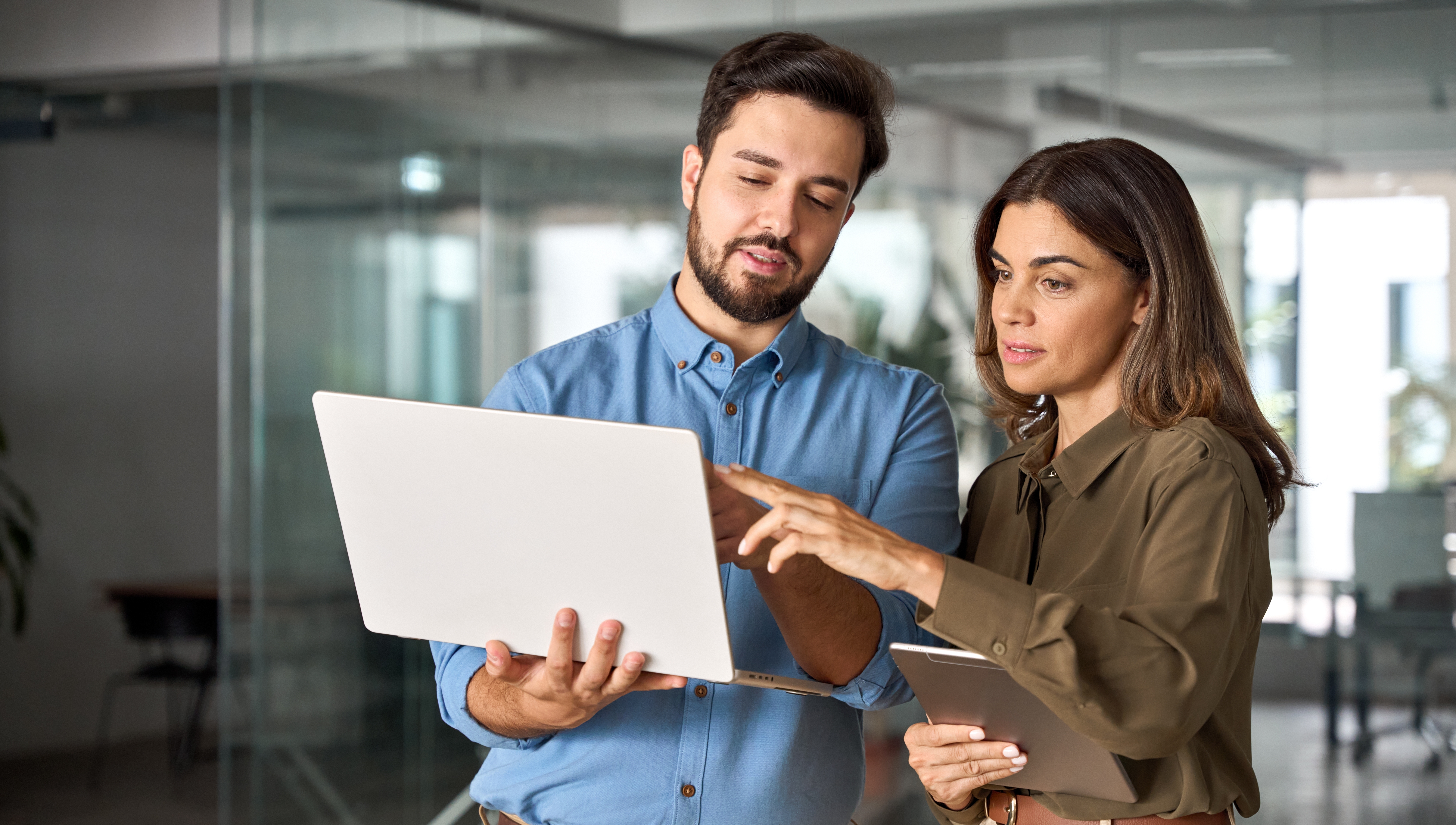
AI models benefit from a holistic view of data, which enables them to make more accurate predictions and decisions. Incomplete or siloed data reduces the value of AI initiatives and creates inefficiencies. Thus, organizations must establish data integration processes that provide clean, integrated, and accessible data for AI systems.
Investing in modern data management and integration tools can simplify this process. These tools ensure that data from different systems is seamlessly merged, cleaned, and transformed for AI consumption. The right tools and technologies ensure that AI initiatives benefit from high-quality, unified data.
The Strategic Importance of Data Quality in AI Initiatives
Organizations must prioritize data quality as part of their broader digital strategy to leverage AI's full potential. Data is essential as a strategic asset, and the quality of that data directly impacts the effectiveness of AI initiatives. Organizations can create a data-driven culture that fosters success across all AI-related projects by aligning data quality initiatives with business goals.
AI initiatives are not just about implementing technology—they are about transforming business processes and delivering value. Organizations that recognize the strategic importance of high-quality data will be better positioned to achieve long-term success. Prioritizing data quality ensures that AI systems can drive innovation and sustainably improve business outcomes.
Furthermore, adopting a strategic approach to data quality allows organizations to scale their AI initiatives. As business needs grow and evolve, ensuring that data quality remains consistent will enable AI systems to adapt and deliver value. Organizations must regularly assess and improve data quality to ensure the success of their AI initiatives.
Partner with Experts to Unlock the Full Potential of Your AI Initiatives
Quality data is at the core of every successful AI initiative. By focusing on data integrity, governance, and accessibility, organizations can ensure that their AI systems operate effectively and provide actionable insights. This leads to enhanced decision-making, improved operational efficiency, and greater business success.
At MSSBTA, we understand the importance of data quality in AI initiatives. Our expertise in digital transformation and IT advisory services can help your organization ensure that your AI initiatives are built on a strong foundation of reliable, high-quality data. Contact us today to learn how we can help you optimize your data and unlock your business's full potential with AI.
Comments