AI integration reshapes industries, but success hinges on a robust data infrastructure. Quality data systems enhance AI’s ability to deliver insights, streamline processes, and drive innovation. However, AI initiatives may fall short without evaluating and optimizing your existing infrastructure. Understanding the critical elements of your data infrastructure prepares your organization for seamless AI adoption, empowering teams to maximize AI's potential.
Evaluating your infrastructure for AI readiness involves examining data quality, accessibility, and compatibility. Each component affects how well AI processes information, makes predictions, and supports business objectives. By carefully assessing these areas, organizations can identify and resolve potential roadblocks before they hinder AI progress. A proactive approach to infrastructure evaluation ultimately leads to more reliable and impactful AI results.
Assessing Data Quality for Accurate AI Integration
Data quality directly impacts the success of any AI integration. High-quality data enables AI algorithms to make accurate predictions, while poor data quality can lead to unreliable outcomes. Before embarking on AI initiatives, organizations should ensure data is clean, complete, and up-to-date. This attention to data quality also forms the foundation for trustworthy AI insights.
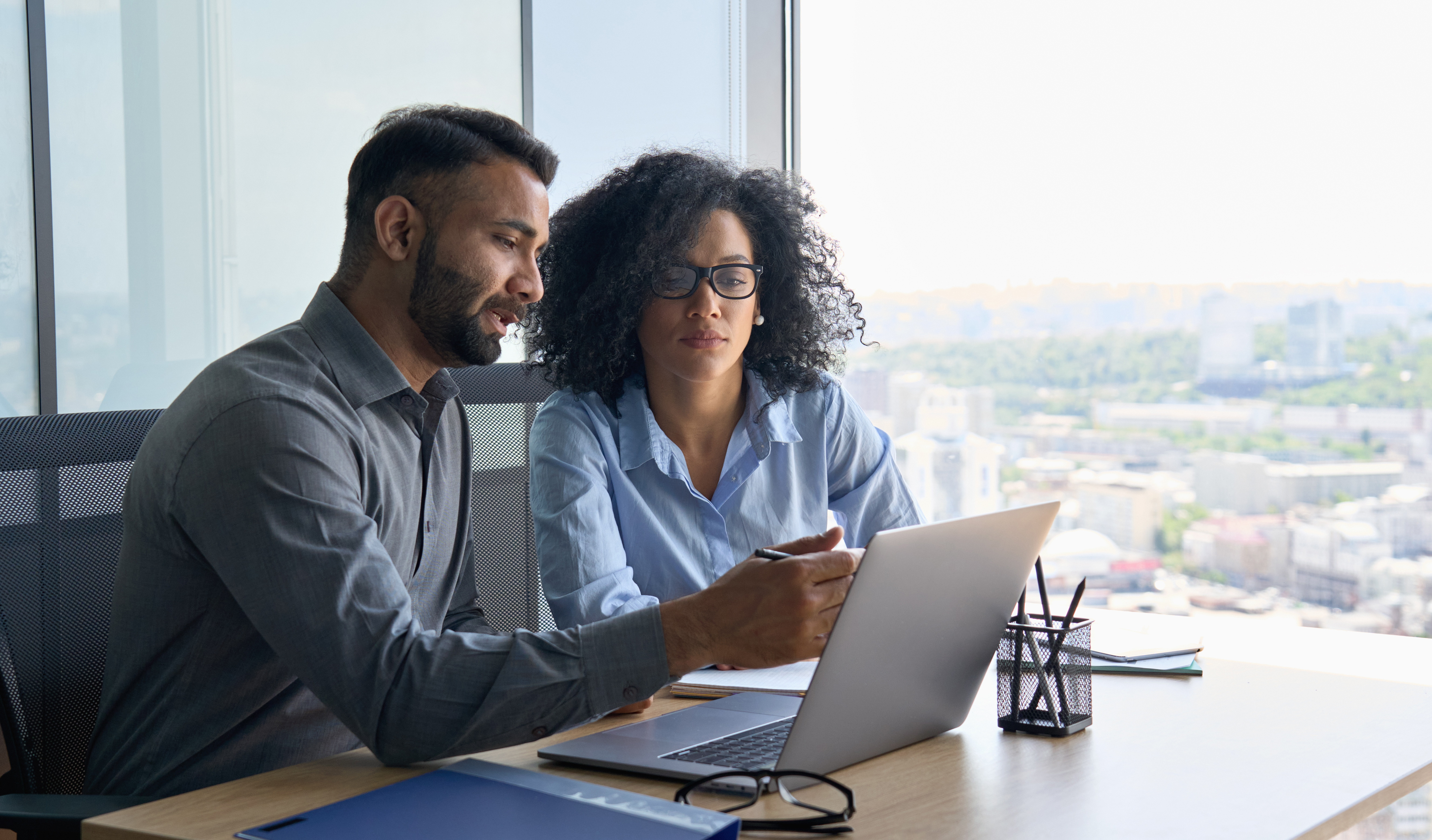
Data quality issues often arise from inconsistent entries, duplicate data, or outdated information. By establishing data governance practices, businesses can create standards for data integrity, reducing the risk of errors that compromise AI performance. Moreover, implementing automated data-cleaning processes can further ensure data remains accurate and ready for analysis.
Regular audits are essential to maintaining data quality. Audits allow organizations to detect and resolve inconsistencies, ensuring AI systems can rely on accurate, actionable information. Organizations prioritizing data quality pave the way for AI tools that deliver dependable, high-value insights.
Optimizing Data Accessibility for Efficient AI Integration
For AI systems to work effectively, data must be readily accessible to authorized users and applications. Limited data access can bottleneck AI processes, slowing analysis and delaying insights. Evaluating your current data access controls is essential to removing barriers and facilitating smooth AI workflows. When data is readily available, AI systems can operate with greater speed and efficiency.
Data accessibility also involves centralizing data sources. Disconnected data silos hinder AI’s ability to draw comprehensive insights. By unifying data sources into a single, accessible platform, organizations enable AI tools to access a holistic view of information, resulting in more accurate analyses.
Ensuring secure access is just as critical. Implementing role-based access controls (RBAC) ensures that only authorized personnel can access sensitive data, reducing security risks while promoting efficient data sharing. Optimizing accessibility without compromising security strikes the right balance for effective AI integration.
Establishing a Data-Driven Culture for AI Success
Effective AI integration requires more than technical readiness; it depends on a data-driven culture where employees recognize the data’s value. Encouraging teams to rely on data insights promotes more informed decision-making and facilitates AI adoption. When data-driven thinking permeates an organization, it fosters an environment where AI initiatives can thrive.
Training programs that promote data literacy help employees understand and trust data analytics. When team members are comfortable interpreting data, they’re more likely to embrace AI tools that enhance their workflows. Organizations prioritizing data literacy create a workforce better prepared to engage with AI.
Leaders can also set an example by using data to drive decisions and promote transparency around AI objectives. When employees see data-driven approaches in action, they’re more likely to adopt similar practices. A data-driven culture supports AI integration and contributes to a more agile, innovative organization.
Enhancing Data Security for Trustworthy AI Outputs
AI relies on large datasets, prioritizing data security in AI integration. Without robust security measures, sensitive information can be at risk, leading to potential breaches and loss of stakeholder trust. Implementing advanced security protocols ensures that data remains protected throughout AI processes, fostering confidence in AI tools.
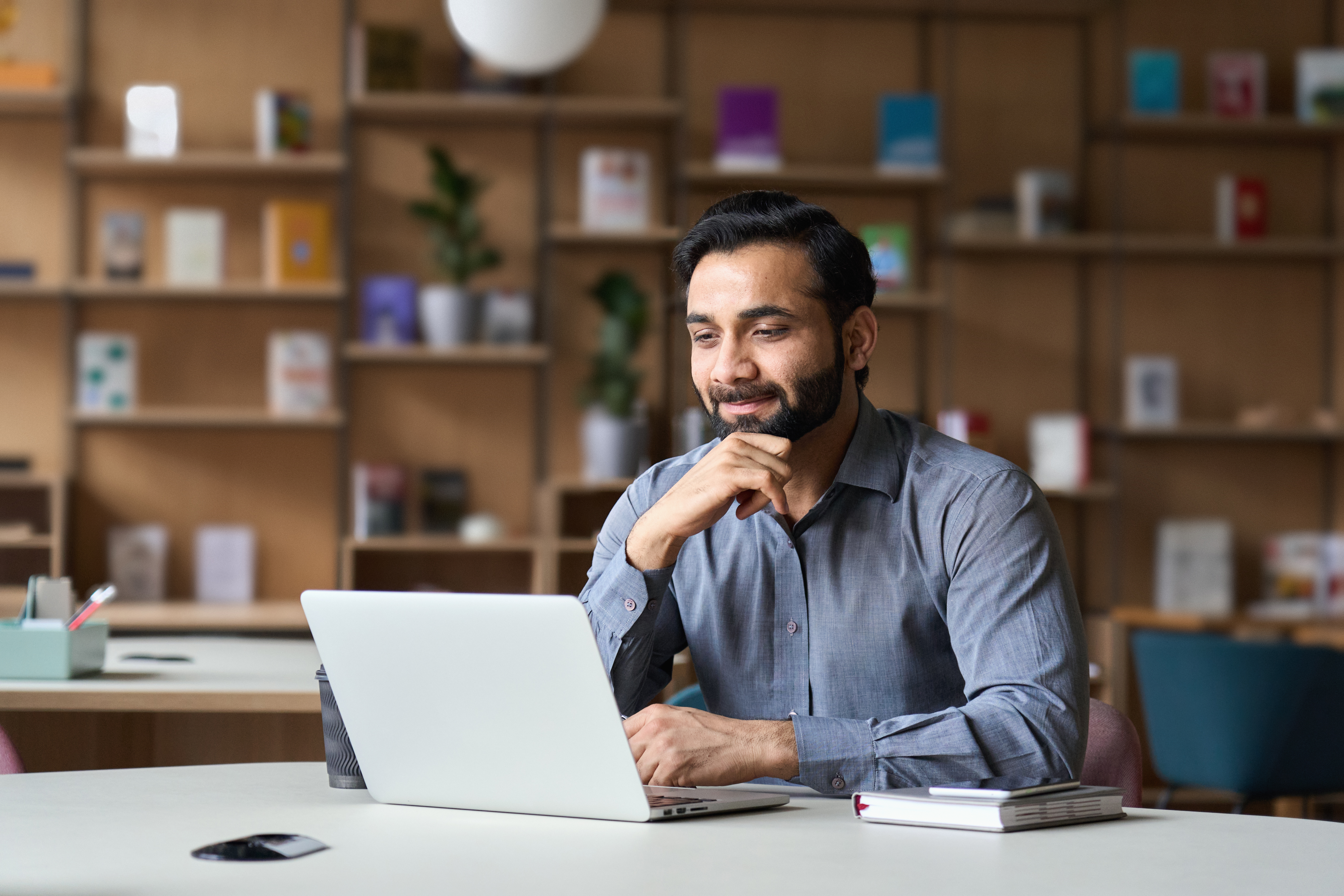
Data encryption safeguards information by transforming it into a secure format, essential for protecting sensitive data. Additionally, secure data storage methods, such as end-to-end encryption, help prevent unauthorized access. Organizations should ensure encryption is applied across all data storage and transfer points to maintain data integrity.
Furthermore, AI’s ability to handle massive datasets means data privacy regulations like GDPR and CCPA must be considered. Compliance with these regulations is essential for maintaining both legal and ethical standards. Robust security protocols and adherence to data privacy standards provide a solid foundation for trustworthy AI integrations.
Ensuring Infrastructure Compatibility for Seamless AI Integration
Compatibility within your infrastructure plays a significant role in the success of AI initiatives. Legacy systems or incompatible software can create obstacles to AI integration, limiting an organization’s ability to leverage AI fully. By assessing compatibility, businesses can identify system updates or replacements needed to support AI technology.
Modern AI solutions often require flexible, scalable infrastructures. Cloud-based environments provide scalability and compatibility that on-premises systems may lack, making them ideal for AI. Organizations should evaluate whether their current infrastructure can support AI processing requirements and consider cloud or hybrid solutions for greater flexibility.
System integration is another consideration. AI systems often require a degree of interoperability with other enterprise applications, such as CRM or ERP platforms. Ensuring these systems work harmoniously allows for a smoother AI integration, improving the flow of data and the accuracy of insights generated.
Scaling Infrastructure for Future AI Needs
AI demands considerable computational power and data storage. As AI grows within an organization, so does the need for scalable infrastructure to handle increased workloads. Evaluating your capacity and planning for scalability ensures that your infrastructure can accommodate future AI developments, allowing AI to evolve with your organization’s needs.
Cloud computing offers flexible scalability, making it easier to adjust resources as needed. By adopting cloud-based solutions, businesses can ensure their infrastructure meets the demands of AI processing without overextending their resources. This adaptability is key to staying competitive in an AI-driven marketplace.
Long-term infrastructure planning also involves regularly reviewing AI needs and updating resources accordingly. As AI applications become more complex, having a scalable infrastructure in place prevents performance issues and minimizes the need for costly overhauls. Building a scalable infrastructure from the outset provides a foundation for sustainable AI growth.
Preparing Your Infrastructure for Effective AI Integration
Evaluating and optimizing your data infrastructure is essential for successful AI integration. By focusing on data quality, accessibility, compatibility, security, and scalability, organizations lay a foundation for AI that delivers value and insights. Building a data-driven culture further supports AI adoption, enabling teams to fully leverage AI’s potential.
For expert guidance on AI integration and to ensure your infrastructure is ready, reach out to MSSBTA today.
Comments