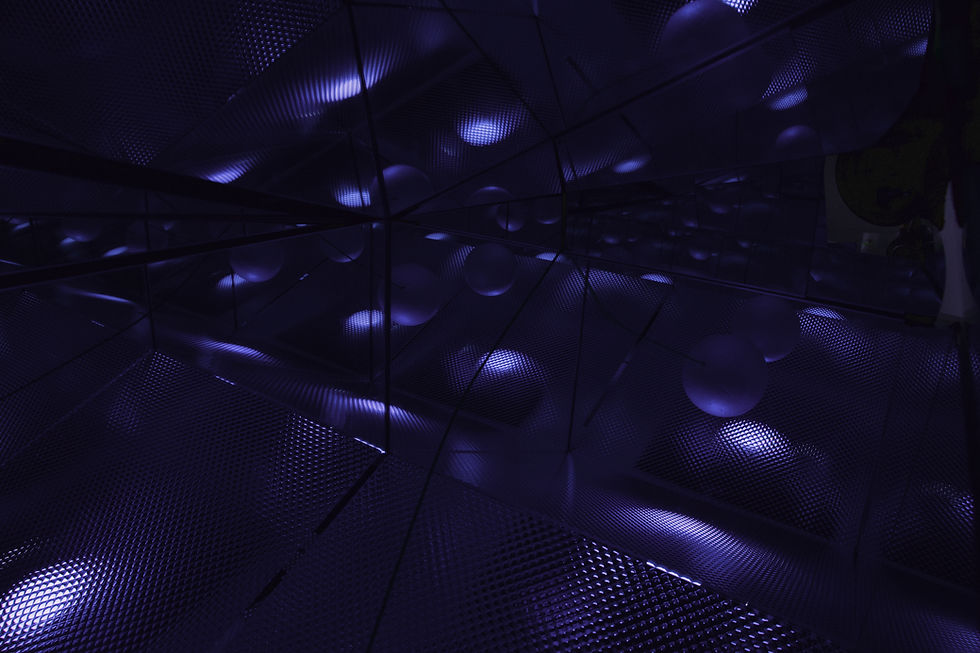
In an era defined by the exponential growth of data and the proliferation of AI, concerns surrounding data privacy, data minimization, data provenance, and data lineage have come to the forefront of organizational priorities. Businesses must navigate the complex landscape of regulatory compliance, ethical considerations, and technological advancements to safeguard data integrity and protect individual privacy. In this article, we'll explore the significance of these concepts and how AI can serve as both a challenge and a solution in ensuring responsible data stewardship.
Data Privacy: Upholding Individual Rights
Data privacy, also called information privacy, refers to the protection of individuals' personal information from unauthorized access, use, and disclosure. With the increase of the creation of personal data, this right and ability to control how this information is created, stored and shared with others has never been more important. With the advent of stringent data protection regulations such as the GDPR (General Data Protection Regulation) and the CCPA (California Consumer Privacy Act), organizations face heightened scrutiny and accountability regarding their data handling practices. Developing AI regulation, such as Europe’s AI Act and the recent US’s White House AI Executive Order also has effects upon data privacy.
AI presents both opportunities and challenges in the realm of data privacy. On one hand, AI-powered data anonymization and encryption techniques can enhance privacy safeguards by minimizing the risk of unauthorized data access. On the other hand, the proliferation of AI-driven analytics and predictive modeling raises concerns about the potential for algorithmic bias and discriminatory outcomes, posing risks to individual privacy rights.
To address these challenges, organizations must prioritize transparency, accountability, and ethical AI practices in their data processing workflows. By implementing privacy-preserving AI technologies such as federated learning, differential privacy, and homomorphic encryption, organizations can uphold individual privacy rights while leveraging the power of AI to drive innovation and value creation.
Data Minimization: Less is More
Data minimization refers to the principle of collecting and retaining only the minimum amount of data necessary for a specific purpose. Less is more and the goal. In an age where data is often touted as the new oil, organizations must exercise caution to avoid indiscriminate data collection and storage practices that can increase the risk of data breaches and privacy violations.
AI can play a pivotal role in facilitating data minimization efforts through techniques such as data anonymization, aggregation, and de-identification. By applying AI-driven data analytics and machine learning algorithms, organizations can extract actionable insights from large datasets without compromising individual privacy or exposing sensitive information.
Furthermore, AI-powered data governance platforms can help organizations enforce data minimization policies by providing visibility into data usage, access controls, and retention policies. By leveraging AI to automate data classification, tagging, and lifecycle management, organizations can ensure compliance with regulatory requirements and minimize the risk of data misuse or unauthorized access.
Data Provenance and Lineage: Tracing the Origins
Data provenance and lineage refer to the ability to trace the origins, transformations, and movement of data throughout its lifecycle. In an increasingly interconnected and data-driven world, maintaining visibility and auditability of data lineage is essential for ensuring data integrity, accountability, and trustworthiness.
AI can enhance data provenance and lineage capabilities by providing automated tools for tracking and documenting data lineage across complex data ecosystems. By leveraging AI-driven metadata management and lineage tracking solutions, organizations can gain insights into data lineage dependencies, data quality issues, and lineage impact analysis.
Furthermore, AI-powered anomaly detection and pattern recognition algorithms can help organizations identify deviations from expected data lineage patterns and detect potential data breaches or unauthorized data access. By proactively monitoring data provenance and lineage, organizations can enhance data governance, mitigate risks, and maintain compliance with regulatory requirements.
Conclusion: Leveraging AI for Responsible Data Stewardship
In closing, the convergence of data privacy, minimization, provenance, and lineage underscores the importance of responsible data stewardship in the age of AI. As organizations grapple with the complexities of managing vast amounts of data while upholding individual privacy rights and regulatory compliance, AI emerges as a powerful ally in addressing these challenges.
By harnessing the capabilities of AI-driven privacy-preserving technologies, data governance platforms, and lineage tracking solutions, organizations can strengthen their data protection strategies, mitigate risks, and build trust with stakeholders. However, it is imperative for organizations to approach AI adoption with caution, ensuring transparency, accountability, and ethical considerations are embedded into AI-driven decision-making processes.
Ultimately, by leveraging AI for responsible data stewardship, organizations can unlock the full potential of their data assets while safeguarding individual privacy rights and maintaining trust in the digital ecosystem. As we navigate the evolving landscape of data privacy and AI, the principles of transparency, accountability, and ethical AI practices will serve as guiding beacons for responsible data stewardship in the years to come.
Commentaires