Is Your Legacy Infrastructure Ready for Agentic AI? Key Considerations for IT Leaders
- Peter Meyers
- 1 day ago
- 5 min read
The rapid evolution of artificial intelligence (AI) has introduced agentic AI systems—autonomous agents capable of performing tasks without human intervention. These systems can potentially revolutionize industries by enhancing efficiency and decision-making processes. However, integrating agentic AI into existing legacy infrastructures presents significant challenges for IT leaders. Ensuring compatibility, data integrity, and security requires meticulous planning and strategic foresight.
Assessing Legacy Infrastructure Compatibility with Agentic AI
Evaluating the compatibility of legacy systems with agentic AI is a fundamental step in the integration process. Legacy infrastructures often operate on outdated technologies that may not support the advanced functionalities of modern AI systems. According to CIO.com, 38% of organizations identify integration complexity as the primary barrier to scaling AI agents. IT leaders must conduct
comprehensive assessments to identify technological gaps and determine the feasibility of integration.
Compatibility issues often arise from disparate data formats and protocols used in legacy systems. Agentic AI requires seamless access to diverse data sources to function effectively. Standardizing data formats and establishing robust data integration frameworks are essential to address these challenges.
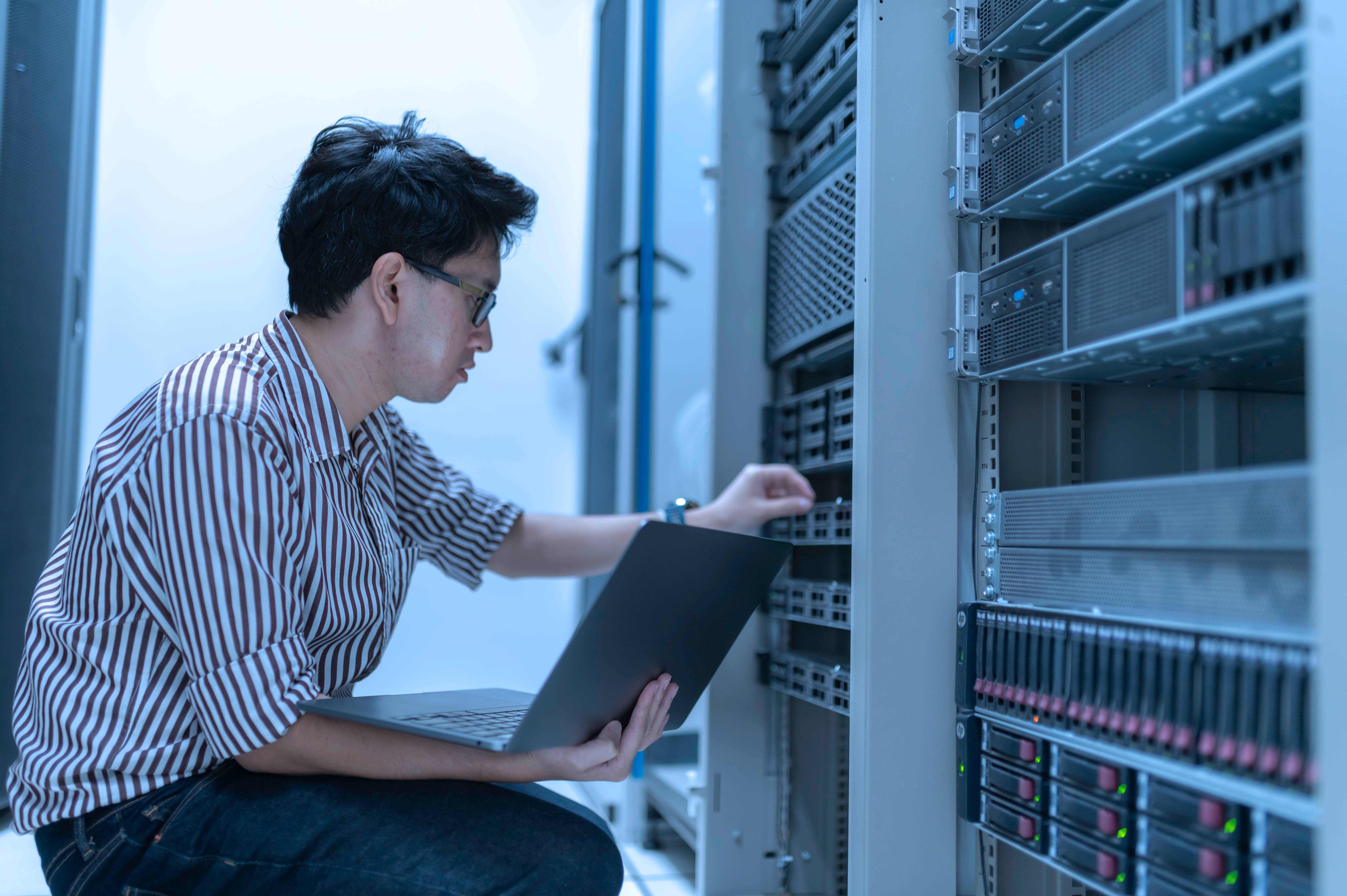
Moreover, the scalability of legacy systems is a concern. Agentic AI applications demand substantial computational resources and the ability to process large volumes of data in real-time. Legacy infrastructures may lack the necessary scalability, hindering the performance of AI agents. Upgrading hardware components and optimizing system architectures are potential solutions to enhance compatibility.
Interoperability between legacy systems and agentic AI platforms is another critical factor. Proprietary technologies and closed architectures in legacy systems can impede seamless integration. Adopting middleware solutions or APIs can facilitate communication between heterogeneous systems, ensuring that AI agents can access and process information effectively.
Data Quality and Integration Challenges for Agentic AI
High-quality data is the cornerstone of effective agentic AI deployment. Legacy systems often contain data silos, inconsistencies, and inaccuracies that can compromise AI performance. Organizations frequently struggle with poor data quality and integration problems within their legacy systems. Addressing these issues is imperative for successful AI integration.
Data silos in legacy infrastructures prevent AI agents from accessing comprehensive datasets, leading to incomplete analyses. Implementing data warehousing solutions or data lakes can consolidate disparate data sources, providing a unified repository for AI processing.
Inconsistencies in data formats and standards pose significant challenges during integration. Agentic AI systems require standardized data to interpret and analyze information correctly. Establishing data governance policies that enforce uniform data standards across the organization is essential. These policies ensure that data collected from various sources adheres to predefined formats, facilitating seamless integration.
Data cleansing is a critical process in preparing legacy data for AI applications. Removing duplicates, correcting errors, and validating data accuracy are necessary steps to enhance data quality. Automated data cleansing tools can streamline this process, reducing the required manual effort and minimizing human error risk.
Real-time data processing capabilities are vital for autonomous agentic AI systems. Legacy infrastructures may lack the ability to handle real-time data streams, limiting the responsiveness of AI agents. Upgrading system architectures to support real-time data ingestion and processing is necessary to fully leverage agentic AI's capabilities.
Finally, ensuring data security during integration is paramount. Agentic AI systems often require access to sensitive information, raising concerns about data breaches and unauthorized access. Implementing robust security protocols, such as encryption and access controls, protects data integrity and maintains compliance with regulatory standards.
Security and Compliance Considerations
Integrating agentic AI into legacy systems introduces new security and compliance challenges. Autonomous AI agents accessing sensitive data can increase the risk of privacy violations and cyber threats. According to Signal President Meredith Whittaker, agentic AI systems performing tasks without human input pose significant privacy risks. IT leaders must proactively address these concerns to safeguard organizational assets.
One primary security concern is the potential for AI agents to access and misuse sensitive information. Implementing strict access controls ensures that AI systems only retrieve data necessary for their functions. Regular audits and monitoring of AI activities can detect anomalies and prevent unauthorized data usage.
Compliance with regulatory standards is another critical consideration. Industries such as finance and healthcare are subject to stringent data protection laws that govern how information is stored and processed. Ensuring that agentic AI systems adhere to these regulations is essential to avoid legal repercussions. Collaborating with legal and compliance teams during integration helps identify and mitigate potential compliance issues.
The complexity of legacy infrastructures can introduce vulnerabilities that malicious actors may exploit. Thorough security assessments identify weaknesses within the system architecture. Patching outdated software, updating security protocols, and implementing intrusion detection systems enhance the security posture of legacy systems.
Change Management and Organizational Readiness
The successful integration of agentic AI into legacy infrastructures extends beyond technical considerations; it requires comprehensive change management strategies. Organizational readiness plays a pivotal role in adopting AI technologies.
Building employee awareness and understanding of agentic AI is the first step toward fostering acceptance. Educational programs and workshops can demystify AI technologies, highlight their benefits, and address common misconceptions. Engaging employees in discussions about AI integration encourages a culture of openness and collaboration.
Leadership commitment is crucial in driving AI initiatives. Leaders must articulate a clear vision for AI integration, aligning it with organizational goals and values. Demonstrating support and involvement from leadership fosters confidence and motivates employees to embrace the changes associated with AI adoption.
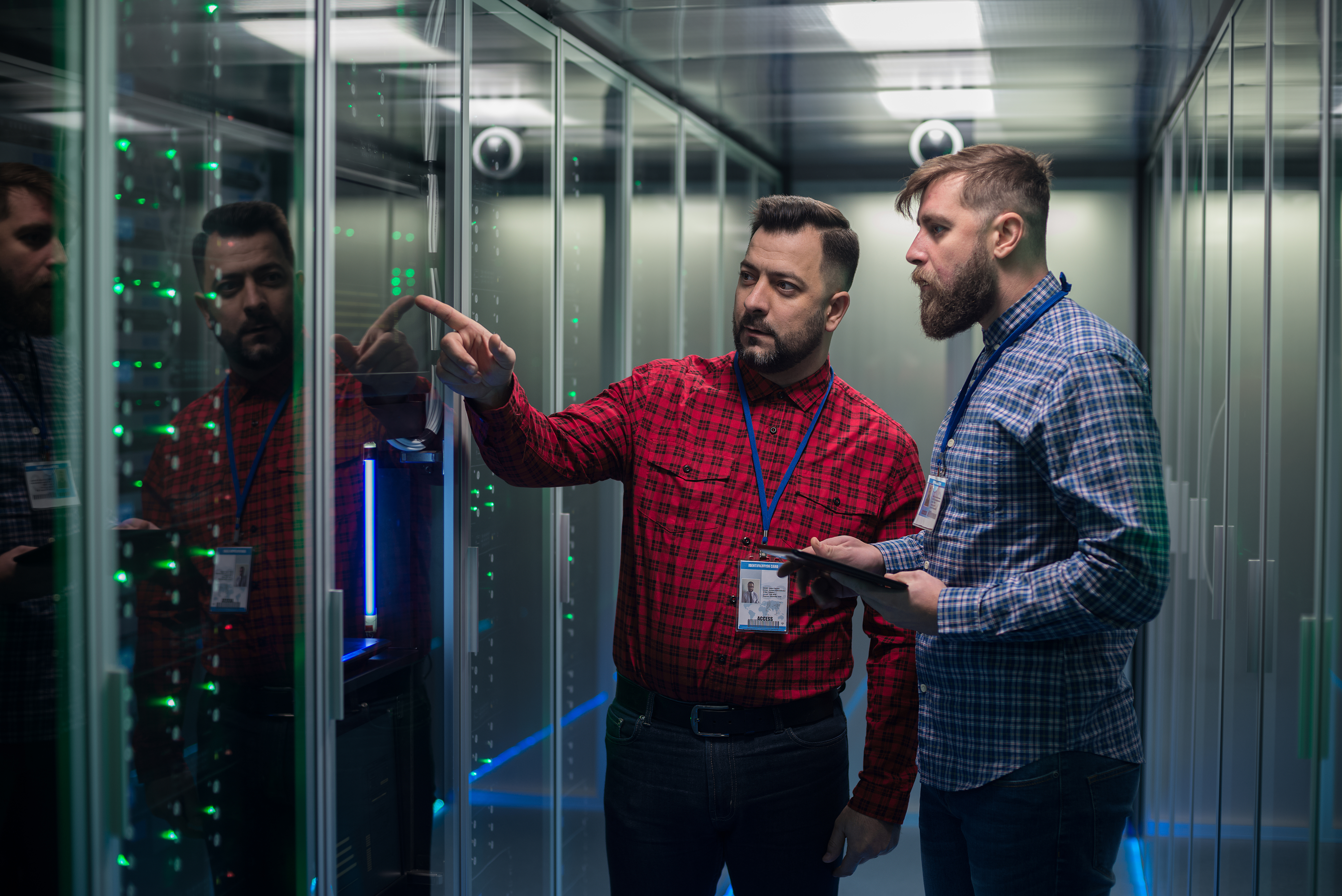
Identifying and empowering AI champions within the organization can facilitate smoother transitions. These individuals can serve as advocates, providing guidance and support to their peers during the integration process. Their firsthand experiences and insights can address concerns and promote a positive attitude toward AI technologies.
Communication is a vital component of change management. Transparent and consistent communication strategies inform employees about AI integration efforts' progress, challenges, and successes. Providing platforms for feedback allows employees to voice their opinions and contribute to the continuous improvement of AI initiatives.
Strategic Planning and Future-Proofing Your Infrastructures
Integrating agentic AI into legacy infrastructures necessitates strategic planning to ensure long-term success and adaptability. IT leaders must develop comprehensive roadmaps that align AI initiatives with organizational objectives and future technological advancements. They must also proactively plan for AI evolution to avoid costly overhauls and disruptions.
Developing a phased implementation approach minimizes risks associated with AI integration. Pilot programs allow organizations to assess AI system performance within controlled environments before full deployment.
Investment in scalable infrastructure ensures that AI implementations remain viable as technology advances. Legacy systems must be modernized to accommodate the computational demands of agentic AI. Cloud computing, edge computing, and hybrid architectures provide scalable solutions that enhance AI system efficiency. Adopting flexible infrastructure models prevents obsolescence and supports future AI advancements.
Collaboration with AI vendors and technology partners facilitates seamless AI integration and ongoing innovation. Partnering with experts in AI development ensures that organizations stay ahead of emerging trends. Establishing long-term relationships with AI providers enhances access to the latest advancements, best practices, and support services. Strategic partnerships accelerate AI adoption while mitigating potential risks.
Finally, continuous monitoring and performance evaluation optimize AI operations. Establishing key performance indicators (KPIs) and benchmarking AI effectiveness ensures that AI systems align with business goals. Regular assessments identify areas for improvement and enable adaptive strategies. A proactive approach to AI management maximizes long-term value and operational efficiency.
Future-Proof Your IT Infrastructure for Agentic AI
Agentic AI presents immense opportunities for organizations to enhance automation, efficiency, and decision-making. However, integrating AI into legacy infrastructures requires a structured approach that addresses compatibility, data quality, security, change management, and future scalability. IT leaders must adopt a proactive strategy to modernize their systems while ensuring compliance and security.
MSSBTA specializes in guiding organizations through complex IT transformations, helping IT leaders confidently navigate AI adoption. Our expertise in system integration, change management, and data governance ensures a seamless transition to AI-driven operations. Contact MSSBTA today to assess your IT infrastructure’s readiness for agentic AI and future-proof your organization’s digital strategy.
Коментарі