Artificial intelligence (AI) is transforming industries, but its rapid adoption raises significant concerns about ethics in AI. Organizations must balance innovation with responsibility to avoid harm. Ethical AI implementation ensures fairness, transparency, and accountability while driving business value.
The ethical challenges of AI are multifaceted, spanning bias, privacy, and accountability. Without proper governance, AI systems can perpetuate discrimination or violate user trust. Ethical implementation requires aligning technology with organizational values and societal norms.
Aligning AI Strategy with Ethical Principles
AI strategy must prioritize ethics in artificial intelligence from inception. Ethical alignment ensures AI systems reflect organizational values and societal expectations. This requires integrating ethical considerations into every stage of AI development. A well-defined strategy mitigates risks and builds trust with stakeholders.
Organizations should establish ethical guidelines tailored to their industry. These guidelines must address fairness, transparency, and accountability. For example, AI systems in healthcare must prioritize patient privacy and data security. According to studies, ethical AI in healthcare can improve outcomes while safeguarding patient rights. A clear framework ensures consistency across projects.
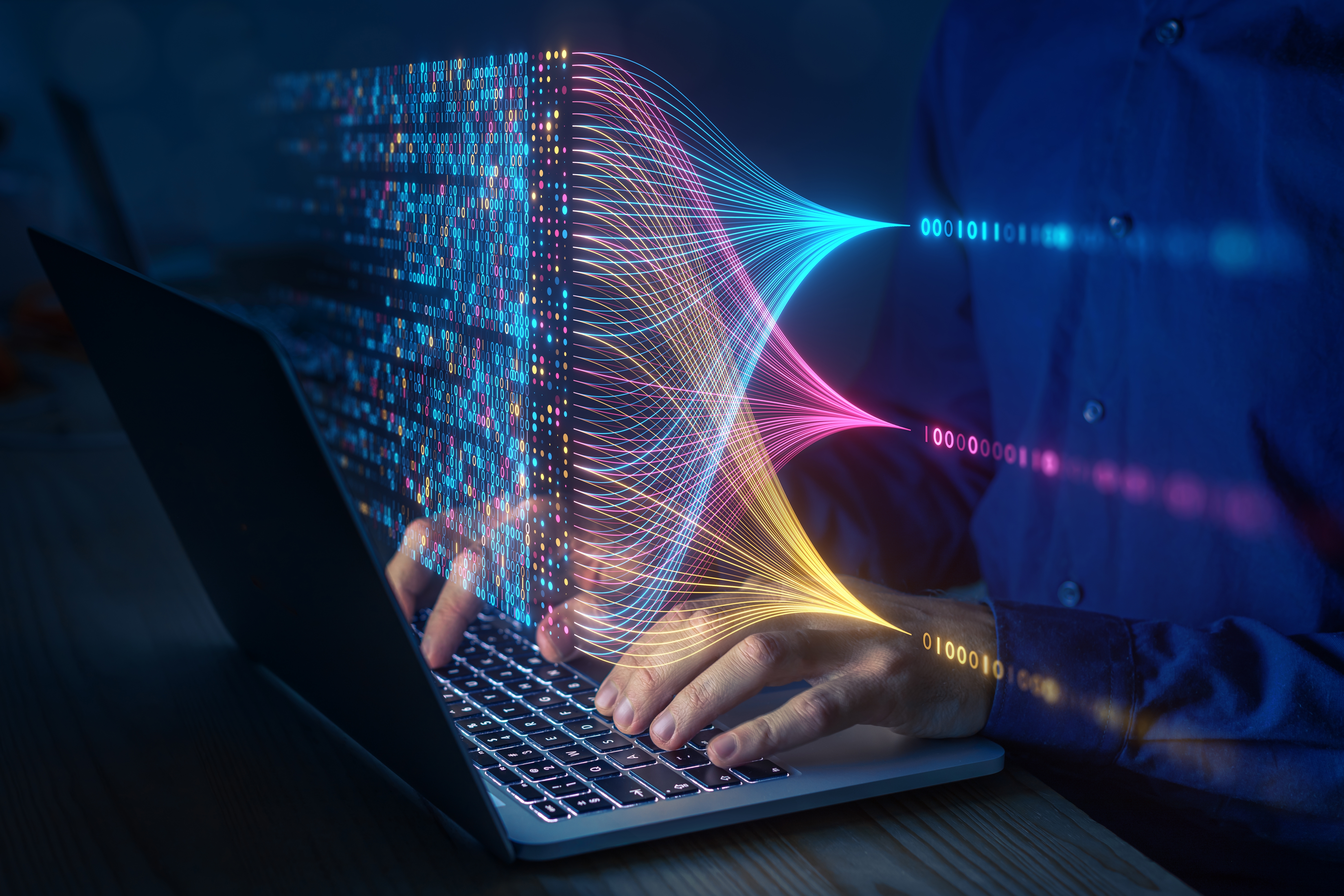
Ethical AI strategy also involves stakeholder engagement. Collaborating with employees, customers, and regulators fosters trust and accountability. According to statistics, 73% of executives believe ethics guidelines in artificial intelligence are crucial, but only 6% have developed them for their organizations. Stakeholder input helps identify potential ethical risks early. This collaborative approach ensures AI systems align with diverse perspectives.
Implementing ethical AI requires robust governance structures. Governance frameworks define roles, responsibilities, and decision-making processes. They ensure accountability at every stage of AI implementation. Effective governance minimizes ethical risks and enhances system reliability.
Training and education are critical for ethical AI adoption. Employees must understand ethical principles and their application in AI workflows. Regular training programs foster a culture of ethical awareness. According to IBM, 2.9% of all AI spending in 2022 was allotted to ethical guidance, which grew to 4.6% in 2024. This empowers teams to make informed decisions during implementation.
Finally, organizations must continuously monitor and evaluate AI systems. Regular audits identify ethical gaps and ensure compliance with guidelines. Monitoring also helps adapt to evolving ethical standards.
Mitigating Ethics Bias in Artificial Intelligence
Bias in AI systems can perpetuate discrimination and undermine trust. Mitigating bias is a cornerstone of ethics in artificial intelligence. Organizations must ensure AI systems treat all users fairly. According to previous studies, 38.6% of AI systems exhibit some form of bias.
Data quality is the foundation of unbiased AI. Biased training data leads to biased outcomes. Organizations must curate diverse and representative datasets. According to Chapman University, AI bias issues stem from unrepresentative data. This reduces the risk of discriminatory AI behavior.
Algorithmic fairness is equally important. Algorithms must be designed to minimize bias and ensure equitable outcomes. Techniques like fairness constraints and adversarial debiasing can help. Human oversight is essential for bias mitigation. AI systems should not operate in isolation. Human reviewers can identify and correct biased outcomes. According to an IAEME study, human-in-the-loop systems reduce bias by as much as 71%. This ensures AI decisions align with ethical standards.
Transparency is key to addressing bias. Organizations must document AI decision-making processes. Clear documentation helps stakeholders understand how decisions are made. Regular bias audits are necessary for ongoing fairness. Audits identify and address biases that emerge over time, ensuring AI systems remain ethical throughout their lifecycle.
Ensuring Ethics Transparency and Explainability in Artificial Intelligence
Transparency is a cornerstone of ethics in artificial intelligence. Stakeholders must understand how AI systems make decisions. Explainable AI (XAI) techniques provide insights into algorithmic processes.
Organizations should prioritize explainability in AI design. Complex models like deep learning can be opaque. Techniques like LIME and SHAP help interpret model outputs. According to Carnegie Mellon University, while these methods make AI decisions more understandable, they do not fully elucidate how the processes work.
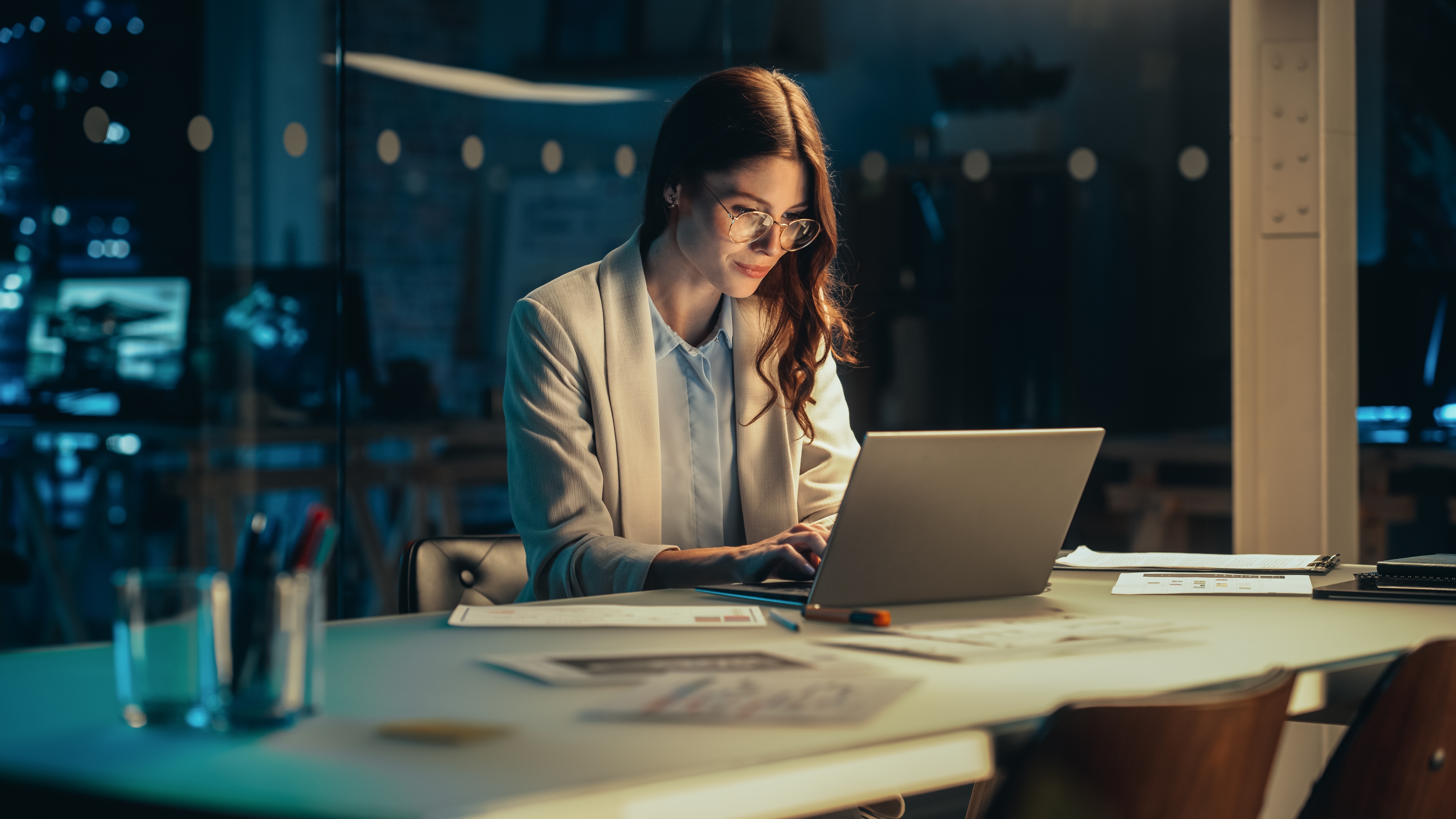
Documenting AI processes is critical for transparency. Organizations must maintain detailed records of data sources, algorithms, and decision criteria. This documentation supports audits and regulatory compliance. According to studies, lack of proper documentation for AI systems is a barrier for adoption.
User communication is another aspect of transparency. Organizations must explain AI decisions to end-users in clear terms, ensuring users understand and trust AI outcomes.
Regulatory compliance further reinforces transparency. Laws like the EU AI Act require explainability for high-risk AI systems. Compliance ensures organizations meet legal and ethical standards. According to EY, 60% of organizations are updating their AI systems to comply with new regulations, reducing the risk of penalties and reputational damage.
Safeguarding Privacy in the Implementation of Ethics in Artificial Intelligence
Privacy is a critical ethical concern in AI implementation. AI systems often process sensitive personal data, and organizations must ensure data is collected, stored, and used responsibly. According to a report by Data Dynamics, 74% of consumers prioritize privacy in AI systems. This protects user privacy and builds trust.
Data minimization is a key principle for privacy protection. Organizations should collect only the data necessary for AI tasks, reducing the risk of misuse and breaches. Encryption and anonymization enhance data security. Sensitive data must be protected during storage and transmission, preventing unauthorized access and misuse.
User consent is essential for ethical data use. Organizations must inform users about data collection and usage. Precise consent mechanisms ensure users retain control over their data. According to a study, 94% of users want control over their data, which fosters trust and compliance.
Regular privacy audits are necessary for ongoing protection. Audits identify vulnerabilities and ensure compliance with privacy standards. This proactive approach minimizes risks and enhances data security. According to a 2023 report by CFO.com, 70% of organizations conduct annual privacy audits. It also supports ethical AI implementation.
Organizations must also prepare for data breaches. Incident response plans ensure swift action in case of breaches. This minimizes harm and maintains user trust.
Build Ethics Into Your AI
Ethics in artificial intelligence are complex but essential endeavors. Organizations must align AI strategy with ethical principles, mitigate bias, ensure transparency, safeguard privacy, and build accountability. These steps ensure AI systems are fair, trustworthy, and compliant with regulations.
Ready to navigate ethics in artificial intelligence with confidence? Partner with MSSBTA to implement AI systems that align with your values and drive sustainable growth. Contact us today to learn more about our expertise in ethical AI implementation.
Commentaires