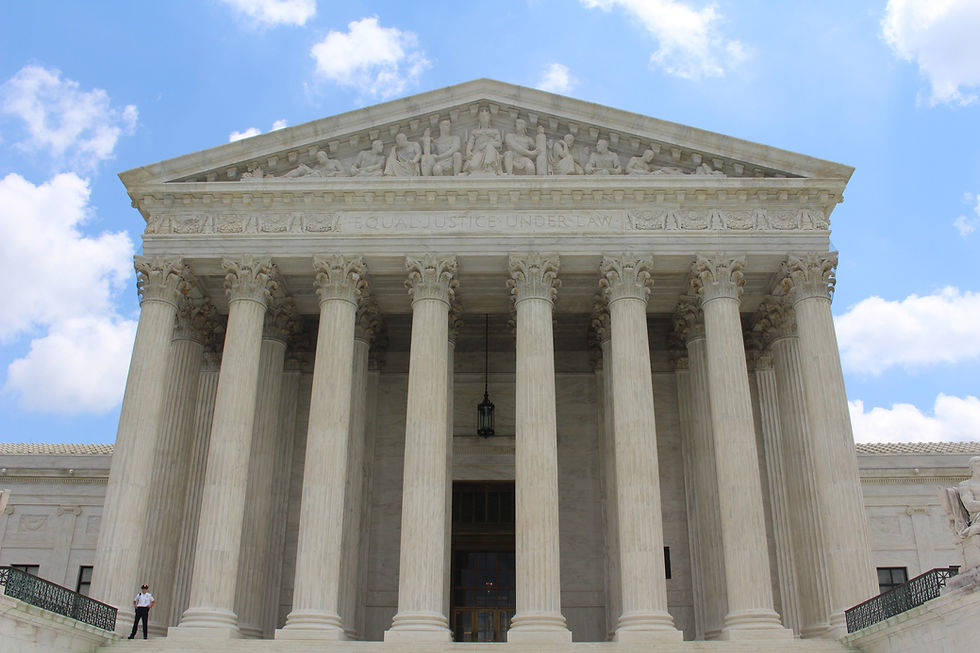
Data governance is a foundational component of an organization's digital roadmap and AI strategy, transcending the technical aspects of data management to create a strategic framework of policies, standards, and procedures. This framework ensures consistent and secure data management across the organization, aligning data with business needs, supporting strategic initiatives, and enhancing capabilities. In the context of the public sector, effective data governance is not just crucial but also responsible for adapting to digital advancements, complying with data privacy regulations, and mitigating risks, particularly when integrating AI technologies. It requires a strong commitment from all involved, especially Public Sector CIOs.
The Role of Data Governance in AI
Data is a critical asset; effective management can provide valuable insights and a competitive edge. As businesses increasingly adopt data-driven strategies, data governance becomes pivotal for ensuring AI initiatives are supported by accurate, consistent data policies. This enables data-driven insights to enhance stakeholder services, optimize operations, and address industry disruptors. For public sector CIOs, data governance ensures that AI applications are built on reliable and compliant data.
Key Components of a Successful Data Governance Strategy
Establishing a robust data governance strategy involves several key components:
Enterprise Governance: Align data governance with your organization’s objectives and overall enterprise governance. Set clear goals that support fiscal management, transparency, accuracy, and compliance with financial regulations.
Data Ownership & Stewardship: Designate data owners and stewards to maintain data quality and compliance. This fosters collaboration between business and IT, ensuring practical and enforceable data policies. For example, assign roles for managing data related to property tax assessments or budgeting.
Policies & Procedures: Develop a comprehensive framework of policies, procedures, and standards for data collection, storage, access, and protection. Create specific policies for handling tax records, financial reports, and audit trails to ensure compliance with regulations.
Policy Development: Define data access controls, usage guidelines, and retention protocols.
Compliance and Security: Implement encryption and access controls to protect sensitive financial data.
Data & Quality Management: Implement processes and technologies for managing data quality, including automated data validation and error-checking systems.
Data Literacy & Culture: Promote a data-driven culture by providing training on data accuracy and its importance in financial reporting and decision-making.
Data Leadership: Ensure strong leadership and executive support for data governance initiatives. This includes driving data integrity and securing support from other county departments.
Continuous Monitoring and Improvement
Regular assessment and enhancement of data governance practices are essential. Utilize key performance indicators (KPIs) to demonstrate the value of data governance, such as accuracy in financial forecasts and efficiency in tax collection processes. Regular audits, feedback loops, and performance metrics will help maintain and improve the governance framework.
Data Governance Policy and Management of Data Construct/Ownership from an AI Standpoint
Data Governance Policy Development:
Policy Framework: Develop policies integrating AI considerations, such as data quality standards for AI training datasets and compliance with AI regulations.
Compliance with AI Regulations: Ensure policies are updated to comply with evolving AI regulations, including provisions for automated decision-making and AI transparency.
Management of Data Construct/Ownership:
Data Ownership: Assign responsibility for data accuracy, privacy, and security to specific roles or departments. For instance, designate teams to maintain data integrity in AI-driven financial forecasts.
Data Access and Control: Implement access controls to ensure only authorized personnel can interact with sensitive data used in AI models.
Data Provenance: Track data lineage to ensure accuracy and reliability, documenting data sources, transformations, and usage.
Integrating AI with Data Governance:
AI-Specific Data Management: Develop procedures for managing data specific to AI applications, including data preparation, model training, and evaluation.
Ethical Considerations: Address ethical issues related to AI, such as avoiding discrimination or bias in AI algorithms, and integrate these guidelines into the overall data governance framework.
Future Trends and Developments
The rise in data volume and variety and advancements in AI, machine learning, and cloud computing are transforming data governance. AI can assist in managing large data volumes and enhancing data discovery and inventorying. Staying abreast of these trends is crucial for aligning successful data governance with enterprise frameworks and ensuring your data practices support business value.
Key Takeaways
Evaluating and enhancing your data governance program for public sector CIOs is essential as you embark on AI initiatives. Ensure your data governance aligns with your business strategy, secures strong leadership, and remains adaptable to evolving data landscapes. Effective data governance should facilitate your AI journey, driving strategic value and supporting data-driven decision-making while mitigating risks.
Commentaires